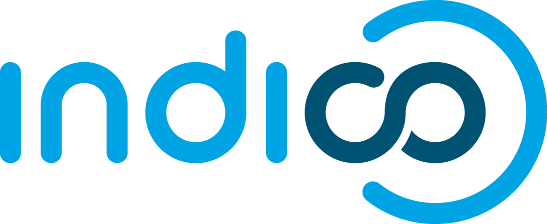
Please log in to access this page.
or change authentication provider
If you do not have an Indico account yet, you can create one here.
Please note that if you have IDEM credentials (which all INAF associated people have, and can be changed or activated here), you must login with LDAP using those credentials (with username of the form name.surname). As such we will not accept registration requests from INAF associated personnel.